New-look Nuance concentrates on conversational AI and biometric security
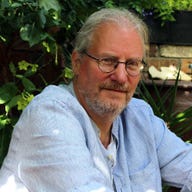
The past year has been an eventful one for Nuance, a company with a rich heritage in OCR and document management, speech recognition, conversational AI and biometrics. In February Nuance sold its document imaging division to Kofax for $400 million, and in October it completed the spin-off of its automotive business into a new public company, Cerence. From now on, Nuance will concentrate on conversational AI and biometrics, particularly in the enterprise customer engagement and healthcare sectors.
ZDNet recently caught up with two executives – Brett Beranek (general manager, security business) and Seb Reeve (director, international go-to-market) – at Nuance's brand-new Soho Square office in London to explore the company's new, more focused, direction.
Brett Beranek, general manager of Nuance's security business.
"We've got a new CEO [Mark Benjamin, appointed in April 2018], and it's good from time to time to have a fresh perspective on a business," said Beranek. "Mark's been laser-focused on making sure that Nuance is on a strong growth path, and has looked at the various divisions and product lines and identified those that are growth vectors for the company. The sale of imaging was part of that — and it was hard for us emotionally to make that change, because back in the 1990s OCR was the genesis of our business."
SEE: How to implement AI and machine learning (ZDNet special report) | Download the report as a PDF (TechRepublic)
"Automotive is slightly different because there's a lot of excitement around the industry, with vendors like Apple, Google and other tech companies in this space. This was a product line that needed very strong investment, so the spin-off was an interesting way of doing that — creating a public company out of that one group," Beranek said.
So what's the relationship between the two companies now?
"We own the majority of the shares, so there's still a very strong tie between Cerence and Nuance. In fact, the technology flows both ways, we source technology off each other, and there are strong personal ties too – I'm in Nuance's Montreal office, and the Cerence office is one floor above us."
How about the R&D function that used to underpin all of Nuance's businesses?
"The technologies and research teams that were focused on our enterprise and healthcare groups are part of Nuance, while those that focused on automotive have shifted to Cerence. But there is some overlap, and that's where we have those existing ties — it's very different to the imaging scenario, which was a clean break. Cerence and Nuance are two separate companies, but partners."
Nuance's imaging business has now been sold and the automotive business spun off. Together, these groups accounted for just under a quarter (23.8%) of the company's 2018 revenue, which was dominated by the healthcare (47.6%) and enterprise (23.3%) divisions.
"Nuance, as it is today, is a much more focused organisation. In the past we had very independent siloed divisions, with a common core set of technologies. Even now, I feel a little awkward talking about 'healthcare' and 'enterprise', because those concepts are quickly disappearing: we've merged R&D groups, marketing and sales organisations, so progressively it really is one unified company without any silos. Now, you could see healthcare as a vertical we're addressing, rather than a separate group within Nuance."
So security, which is your area, now covers everything that Nuance does?
"Correct. Security and biometrics were traditionally within 'enterprise' and we didn't spend too much time talking to our healthcare colleagues. But over the last few weeks I've had more conversations with my healthcare colleagues, and with their customers and partners, than I have in the last seven-and-a-half years. It's been quite transformational — and the R&D for security and biometrics is a shared resource between all groups."
Talking of healthcare, can you summarise Nuance's priorities in this key vertical?
"The big innovation is creating this ambient environment, where we want our systems to be able to — in real time — understand what the patient is saying and what the doctor is saying, and be able to provide real-time advice. For that, you need a whole slew of technologies. Traditionally, a doctor would access the system and dictate what just happened with the patient. Now, there's no overt interaction with a computer: it's just ambiently listening in an examination room or an operating theatre. That's where the 'new Nuance' really shines, because in order to recognise whether the patient or the doctor is speaking, that's where voice biometrics comes in — that's where my discussions have been with my healthcare colleagues. Not only do we need to distinguish between patient and doctor, we also need to know which patient it is and which doctor it is, so that we're updating the correct medical records, and for the AI engine in the background to provide the correct advice."
Healthcare is an area where you can't afford to make mistakes — is it qualitatively different from talking to your bank about a loan, for example?
"Of course the stakes are different, and the context is different, but it's actually surprising how similar the situations are. On the enterprise side, our objective has been to make all the interactions between consumers and enterprises as seamless and human-like as possible — but not to exclude the human from the equation. There are times when it makes sense to speak to a human — when seeking financial advice, for example, as you mentioned. If we can get the AI to augment that experience, to provide real-time advice to the agent or adviser, then we can use the exact same technologies."
So professionals should expect AI to help them with their jobs, rather than threaten to take their jobs?
"This came up at a recent event where we launched all of our solutions in the cloud – including the security and biometrics solution, which we're calling Gatekeeper. The message is that AI is a wonderful technology, and everybody at Nuance is extremely excited about it. But we realise that there are all sorts of limitations to how intelligent AI can be today. So we've taken a different approach to most other vendors in that assisting humans — a doctor or a financial advisor, for example — is where we feel that AI makes the most sense at the moment. It's there to make more intelligent decisions – but as an aid, not as a replacement."
What about menial tasks — the sort of things that computers are traditionally good at?
"These are the types of jobs that AI can handle perfectly, and which we should be automating. It's surprising, today, that a lot of individuals call into their bank to see if a payment has gone through, for example. That's a very simple query that should have a very simple answer — 'yes' or 'no'. This kind of interaction should be automated, but in order to automate those experiences, you need to have intelligence: you need to understand what the customer is requesting and who that customer is, because fraudsters often take advantage of self-service systems."
How is Nuance providing this sort of intelligence?
"A notable addition to the Gatekeeper security package is the Lightning Engine, which combines our AI engines for natural language understanding and voice biometrics to provide a unified experience for the customer. The concept is, whether you're speaking to a voice bot or a virtual assistant, you can explain your intent and simultaneously we're going to validate your identity — it's eliminating an overt identification and verification set. An example I've given is, if you speak to your Mum, you don't start by asking her security questions to validate that she is who she is — your brain is smart enough to work it out. We're replicating that, and have a large multinational bank in the US which has been our beta customer for the Lightning Engine: as you speak to a customer care agent, and explain what you're calling about, in the background our security platform is listening in and validating your identity."
SEE: Nuance Q3 results better than expected
"What they did — what was really innovative — was they used the Lightning Engine in the automated part of the contact centre, the IVR [Interactive Voice Response]. When the caller calls in, the system says 'Thank you for calling, how can I help you today?', just like if you walked into a branch. The caller says 'I have a question about my investments', and the system will, in a single step, recognise why you're calling, validate your identity — authenticating that with biometrics — and then determine 'Is this a query that should be handled in an automated way, by the AI engine, or should it be transferred to a live agent?' There could also be a hybrid version, where the system could decide 'We're going to send this to the automated system, but assisted by a human.' We've talked about having human-based interaction assisted by AI, but this is the opposite: this is an AI experience assisted by a human."
Nuance's cloud-based Lighning Engine combines voice biometrics and natural language understanding (NLU) to authenticate individuals within seconds.
So you need to be pretty foolproof on the voice identification for all this to work...
"It needs to be foolproof — and that's the innovation. In the past, on the voice biometrics side, we needed a good five to ten seconds of speech before we could identify you with an acceptable level of certainty for financial institutions. That doesn't sound like a lot, but if you're speaking to a virtual assistant and say 'send fifty dollars to my Mum', that's maybe two or three seconds of natural speech. As a consumer, I expect the system to understand what I've said without asking me follow-up questions, and I expect it to be done within half a second. That's where the Lightning Engine comes in."
"The reason it's well suited to the cloud is that we can very easily scale up or scale down the processing power. One of the limitations we've seen with customers' on-premise deployments is that they create a plan of how much hardware infrastructure they need, they purchase it, and then they're stuck with that infrastructure for several years. It's a very static system. One of the key advantages of Gatekeeper in the cloud is that cloud hardware can be matched to the highs and lows of demand."
"At Nuance we're also excited about the shift to 5G. We're working with one of the world's largest global telecoms providers to bring speech-enabled biometric security experiences to a whole new level, and 5G is a significant enabler for that. The trifecta of 5G, AI and the cloud is opening up all sorts of possibilities for us."
Can we drill down into some of the components of Nuance's security suite — what is ConversationPrint, for example?
"ConversationPrint is a technology that analyses the vocabulary, grammar and sentence structure that you're using, and is actually a brilliant way to counter deepfakes, for example. During a recent demo we showcased how we can detect the audio portion of a deepfake video – we don't do anything on the video component, but we can say 'that voice, in that video, is a fake'. In our space, the contact centre space, this is an important technology, because we want to make sure that fraudsters can't copy your voice and steal your money."
So at the moment it's not possible to copy someone's voice and use it in a fake online conversation?
"The tools aren't there just yet. There's a company called Lyrebird that creates synthetic voices: they have a web tool where you provide some audio — you need to provide like 31 highly scripted sentences — and it will generate a synthetic voice. How that works is, you need to type the phrase you want the synthetic voice engine to produce, and it takes a couple of seconds to produce that voice."
So it would be difficult to conduct a convincing live conversation in this way?
"It would be very difficult. First of all, we can detect that it's a synthetic voice. One of the easiest ways to understand that is, if I ask you to say 'the sky is blue' a hundred times, and I tell you to say it as consistently as you possibly can, there will still be natural variance — we're human beings, and the voice is infinitely variable. The computer will say the same phrase in the exact same way. Organisations such as Nuance and others that create TTS [text to speech] voices don't create tools that a fraudster could use. When a corporation asks us to create a synthetic voice for a voice assistant, we don't publish the tools. We have a team that scours the internet looking for what fraudsters could use, to make sure we're always several steps ahead."
"When Nuance creates a virtual assistant, we take a professional voice artist and have them read hours and hours of script, giving us all the possible variations we need to create a well-sounding voice. We can still detect those voices, although to the human ear they sound very convincing. I'm less worried about corporations that are protected by our systems because we can implement technologies such as Gatekeeper to prevent attacks, and more worried about fraudsters reaching out to individuals and socially engineering them. The typical example is they call an elderly person and try to convince them they're a relative, and say 'send me money' or 'give me your password'."
What's the next level of sophistication in voice analysis — can you do a behavioural overlay on the normal voice, and tell when people are anxious, stressed or ill, for example?
"That's a very good question. About half the characteristics we measure are behavioural and half are physical. With our latest AI engine we can measure over a thousand characteristics of the voice, which is quite phenomenal. When we first started this journey in the 1990s and created our first voice biometric algorithm, it was less than a hundred characteristics — so that's a huge leap forward. Certain variations of the voice are natural and expected, and a cold is a good example of that: when we see that the sinuses are blocked and show the typical characteristics, we will not fail an individual, because that's something we consider as expected. But we can always build in 'guardrails': if we're seeing high levels of stress that are causing the voice pitch and rhythm to increase, we can generate an alert in those cases."
"Dialect is also in use. Businesses want to personalise the experience for their customers, so you can imagine a customer calling into a contact centre and the automated system says 'how can I help you?' If the system detects a Scottish accent, say, or a Northern Ireland accent, maybe the call is directed to an agent with the same accent. So our security and biometrics platform has also proven useful on the personalisation side."
What about situations where biometrics can be fooled — by identical twins, for example?
Security
"Any biometric system has an error rate, and that error rate is variable. Often you'll see 'our error rate is below one percent'. What does that actually mean? If you take a random fraudster trying to access your account, their probability of success is very very low: they have no biometric overlap with you, and can try infinite attempts but they're not going to get anywhere. However, if you have a twin, their biometric overlap with you is obviously high. Instead of zero percent, their ability to access your account may be five percent, for example. When HSBC's system was accessed by a sibling's voice, it took eight attempts, so what we told HSBC was 'why don't you limit the amount of tries?' And for accounts where there is a twin, you can increase the thresholds."
"So it's definitely not foolproof, but it's significantly better than any other method. The beauty of voice recognition is the wealth of characteristics — we have over a thousand. If you look at this fingerprint reader (I won't name the specific model), it measures about 25 characteristics, while facial recognition is basically measuring the geometric shape — the distance between your eyes, your nose and your mouth. An identical twin will fool facial and fingerprint recognition virtually all the time, but their ability to fool voice biometrics is lower – not impossible, but lower."
Sometimes using voice ID isn't appropriate — perhaps you're in a public place and don't want to conduct a conversation. Are there other biometric avenues Nuance can pursue?
"That's a very strong focus for us, especially for the coming year. We have other biometric modalities in our portfolio: we talked about ConversationPrint, which is actually not dependent on voice – when somebody is speaking we convert the voice to text, and the biometric is on the text. If somebody is typing in online chat, in an email, or with a virtual assistant we can use ConversationPrint to biometrically validate the individual in those text-based interactions. We also have a technology we call Behavioural Biometrics, which measures how an individual interacts with a device – how they're typing, tapping and swiping, how they're holding a phone – in order to validate the individual. Our goal is to deliver that Lightning-type experience, even in non-speech interactions."
What's the state of the art in this area – can you really identify me just by the way I hold a phone?
If all we're seeing is how you're holding a phone, the answer is no. If, on the other hand, you're typing, then the answer is we can validate you with a very high level of certainty – enough that we can authenticate you for a banking transaction."
"I can foresee that, a couple of years down the road, the act of proving who you are will seem completely foreign – 'why isn't it just auto-magically validating my identity?'"
Intelligent engagement
Seb Reeve, director, international go-to-market at Nuance.
Turning from biometrics and security to customer engagement via conversational AI, Seb Reeve (director, international go-to-market), takes up the Nuance story.
"Intelligent engagement is about balancing the competing demands of customers' expectations of simplicity with the business upsides – greater customer loyalty, more revenue, lower costs and so on. Across any industry, that's the equation we put ourselves in the middle of. We're putting AI technologies in there to change the balance: can we make it easier for consumers, and can the cost/revenue pressures be rebalanced? It's AI with a very distinct purpose, is the way I'd put it."
"Much of what we're doing is of course conversational, but we're no longer limited to that. We have an expanded view of what we can use machine learning for – for analytics, for example, so businesses can really understand themselves and their customers, and also for prediction. The important thing is, services and the demands on them are increasingly more contextual and personalised. That's the key: brands not offering such generalised experiences to all of their consumers, but distinctive experiences to specific consumers."
Can you give some 'canonical' examples of analytics and prediction in action?
"Our Pathfinder technology is a good example of the analytics end of things, because what we're doing here is analysing human conversation. It bridges the analytics to conversational AI divide for us: can we use Pathfinder's machine learning to better understand human-to-human conversation, mine its complexity, distill that and then use it as input to teach a machine to have a similar conversation?"
"On the prediction side, we've been working with a number of organisations to figure out where it plays a role. We're working with a very large American retailer, and they're starting to not just ask customers' intent, but, given they have identified themselves in some way, machine learning can be used to spot patterns in the life cycle of the customer – how recently they bought something, what they bought, what kind of product, failure rates for those products, how the repeat buying cycle might work in those environments – and start to model and predict future intent based on prior behaviour."
"So if a customer calls in a certain number of days after buying a specific product that we know would typically confuse them on set-up, instead of saying 'how can we help you?', we can say 'are you calling about the TV you bought a week ago?' Provided the prediction is accurate, that's a lower-effort experience for the consumer."
You've certainly got to get it right, otherwise it would just be irritating...
"You can't play twenty questions with the customer, going through all the things they might be calling about – that's the opposite of a frictionless experience! So how you apply these predictive technologies is extremely important. We've also used it in airlines: there's a high predictability between when your flight is going to be and what your call is likely to be about. In the American airline system, people ask for or expect to be offered upgrades, and within a certain time window a call is exceptionally likely to be about that. So instead of asking 'why are you calling?', the system can say 'your upgrade went through and your flight's on time.' It just cuts across the noise and delivers the information you're likely to want, and then says 'is there anything else you need?' A high proportion of people put the phone down at that point because they're done. These are the kinds of enhancements you can make to standard reactive conversational solutions."
What are the main blockers for businesses wanting to make use of conversational AI and predictive analytics in this way – is it having access to the right data, in the right format?
"It's a great question, because it's one of the more important questions, in the end. Everyone over-rotates on the technology – how does the speech or language understanding really work? – but the reality is these technologies have been around, although improving, for a long time. However, the ability to design these experiences is often under-prioritised – and designing a really slick conversational experience is not easy. Data not only helps to inform the design, but also additional data that's coming in, if operationalised, can improve it over time. Businesses aren't generally well set up to mine this data: having the data isn't a problem, but making it intelligible is the hard part. Doing that not only in a project timeframe to build a bot, but then doing it on a daily, hourly basis to inform a team to improve it – that's challenging."
"This is where the more analytic tools like Pathfinder start to help with that, in concert with other management information and analytic tools – which we have. With consultancy and an underpinning toolset, we provide this design-data-technology link. Getting all three right is important: a lot of providers in this space today offer technology, and that's great, but we sit in the middle of all that and provide value against all three."
RECENT AND RELATED CONTENT
Nuance's Project Pathfinder uses AI to build smarter virtual assistants
Nuance Q3 results better than expected
Nuance sells document imaging business for $400 million
Nuance spins off automotive segment into new publicly traded company